
However, as models become more and more complex (I’m staring at you, deep learning), this kind of analysis becomes impossible.
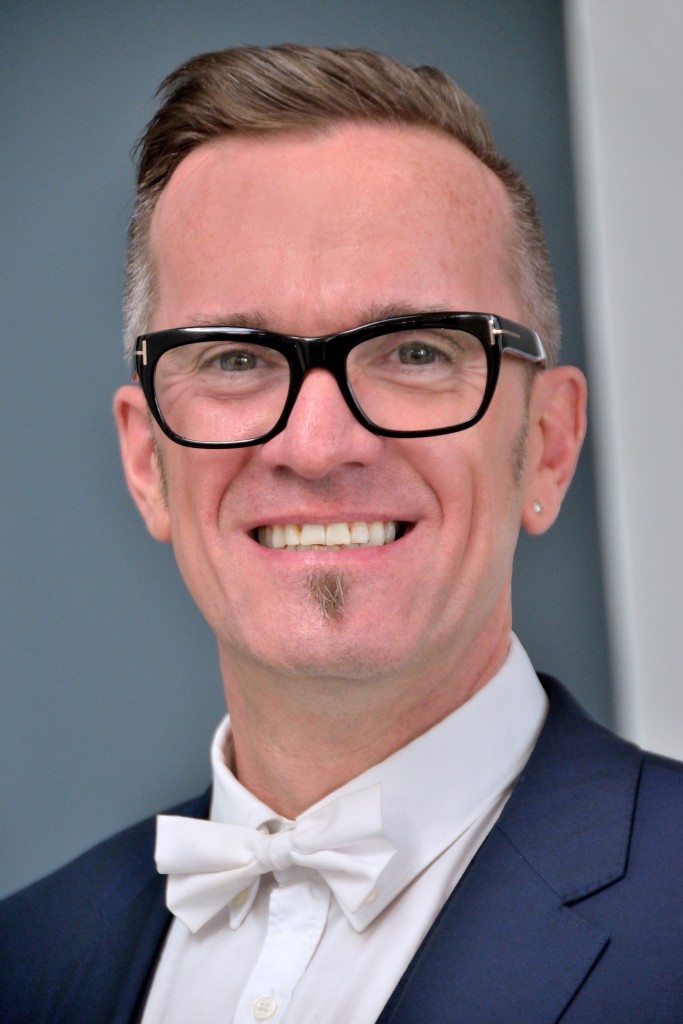
You can then decide whether this process is consistent with your business practices, basically saying: “yes, this is how a human expert would have done it.”
#CLARIFY IMAGE CRACK#
For simple and well-understood algorithms like linear regression or tree-based algorithms, it’s reasonably easy to crack the model open, inspect the parameters that it learned during training, and figure out which features it predominantly uses. Now, let’s discuss the explainability problem. It is thus important for model administrators to be aware of potential sources of bias in production systems. Unfortunately, even with the best of intentions, bias issues may exist in datasets and be introduced into models with business, ethical, and regulatory consequences. Under-representation for such groups could result in a disproportionate impact on their predicted outcomes. In fact, some of these groups may correspond to various socially sensitive features such as gender, age range, or nationality. As the number of classes, features, and unique feature values increase, your dataset may only contain a tiny number of training instances for certain groups. There are many variants of this under-representation problem. In fact, a trivial model could simply decide that transactions are always legitimate: as useless as this model would be, it would still be right 99.9% of the time! This simple example shows how careful we have to be about the statistical properties of our data, and about the metrics that we use to measure model accuracy. fraudulent), there’s a strong chance that it would be strongly influenced or biased by the majority group. Training a binary classification model (legitimate vs. Fortunately, the huge majority of transactions are legitimate, and they make up 99.9% of your dataset, meaning that you only have 0.1% fraudulent transactions, say 100 out of 100,000. Imagine that you’re working on a model detecting fraudulent credit card transactions. They are very real, and their implications can be far-reaching. First, can we ever hope to explain why our ML model comes up with a particular prediction? Second, what if our dataset doesn’t faithfully describe the real-life problem we were trying to model? Could we even detect such issues? Would they introduce some sort of bias in imperceptible ways? As we will see, these are not speculative questions at all. I can’t say it’s perfect but it’s gone from an image that never saw the light of day to one that I keep on my Flickr photostream.Today, I’m extremely happy to announce Amazon SageMaker Clarify, a new capability of Amazon SageMaker that helps customers detect bias in machine learning (ML) models, and increase transparency by helping explain model behavior to stakeholders and customers.Īs ML models are built by training algorithms that learn statistical patterns present in datasets, several questions immediately come to mind. Final step was to downsize to 1000px tall using the bicubic sharpener setting and save as jpeg. These are more aggressive settings than I normally use but actually made much less impact on the final image than Focus Magic. Then I downsized to 2000 pixels tall and ran Nik Output Sharpener at a fairly high setting, with the Focus slider pulled up to 30%. I then cleaned up a few fringes using the clone tool and ran it through Nik Color Efex to correct the colour balance and give the contrast some help. The correction factor was 19, so it’s at the upper end of the corrections that can be done. The sharper version was put into Photoshop CC and first thing I did was run Focus Magic as a layer. The original raw file was converted in Lightroom. So much better than any of the usual sharpening techniques and tools, and so I bought it and use it every now and then when I stuff up a shot that can’t be taken again.
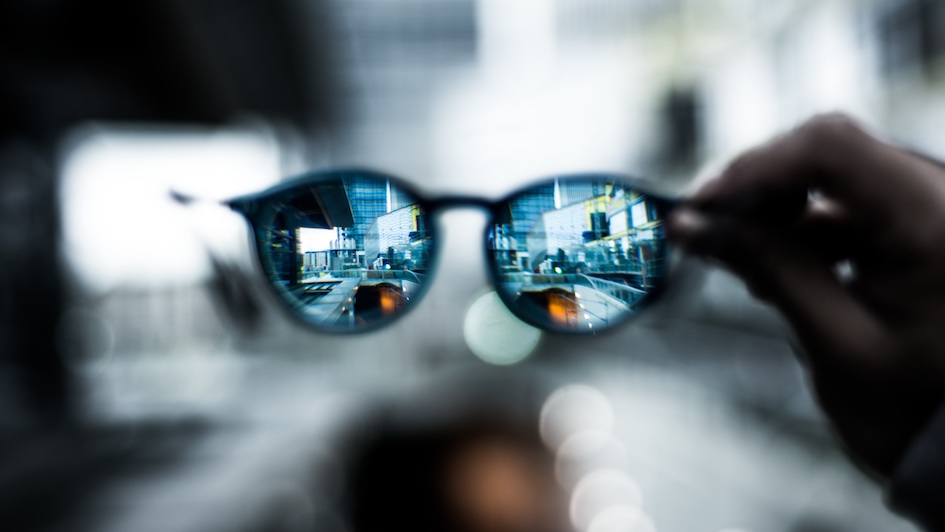
About 6 months later I came across Focus Magic and remembered this blurry image as the perfect test. A quick chimp at the rear screen afterwards didn’t show the focus problem so I thought I was cool and moved on to the next shot.īack at home I saw the problem and had to leave the shot as unsavable. The skater was only above me for a fraction of a second and the camera was set up to ignore such brief changes in focus, so even with an ultra-wide angle lens the shot ended up being focused on the distant sky rather than the guy. The trouble with this was that I completely forgot about how the autofocus would work. So I lay on my back in the bottom of the basin and had the skater come flying over me. I had the idea of shooting from below the skater so I could better see his face. A few years back I was shooting skateboarders at a local park.
